Illegal, Unreported, and Unregulated (IUU) fishing represents a significant global threat to our shared natural resources, undermining the sustainability of fish stocks, damaging ocean ecosystems, and robbing nations of their natural heritage and economic foundation. Nowhere is this challenge more acute than in the Galapagos Marine Reserve (GMR), a biodiversity hotspot of extreme ecological significance. IUU fishing in protected waters, like the GMR, depletes precious marine life and poses a dire threat to the balance of delicate marine ecosystems.
To combat this pervasive threat, Galois is working on new AI-driven technologies designed to augment human efforts in monitoring, detecting, understanding, and acting on illicit transshipment networks and illegal fishing activity in the GMR (where our research focuses) and across the globe. By enabling teaming intelligence in AI co-performers, we are looking to help not only expand human efforts to encompass broad data sets—empowering insights from data sets too big for humans to make sense of on their own—but to provide contextual understanding and explainability to AI outcomes. This will enable human operators to identify patterns and anomalies indicative of IUU fishing, ultimately helping predict illegal activity before it occurs, uncover illicit networks, and protect and restore fragile marine ecosystems.
Dealing with Complex Data
Combating IUU is a complex problem that requires understanding large, diverse sets of data. Our AI-driven approach involves tapping into vast pools of Open Source Intelligence (OSINT) data, including satellite and vessel transponder data provided by Global Fishing Watch (https://globalfishingwatch.org/). All told, we have more than nine billion “tracks” of vessel transponder data—decades of information cataloging the behavior of fishing vessels, “carriers” (boats or ships that transfer illegal hauls from fishing vessels to ports), and “bunkers” (vessels that service and fuel vessels at sea, but often also carry illicit cargo in secret).
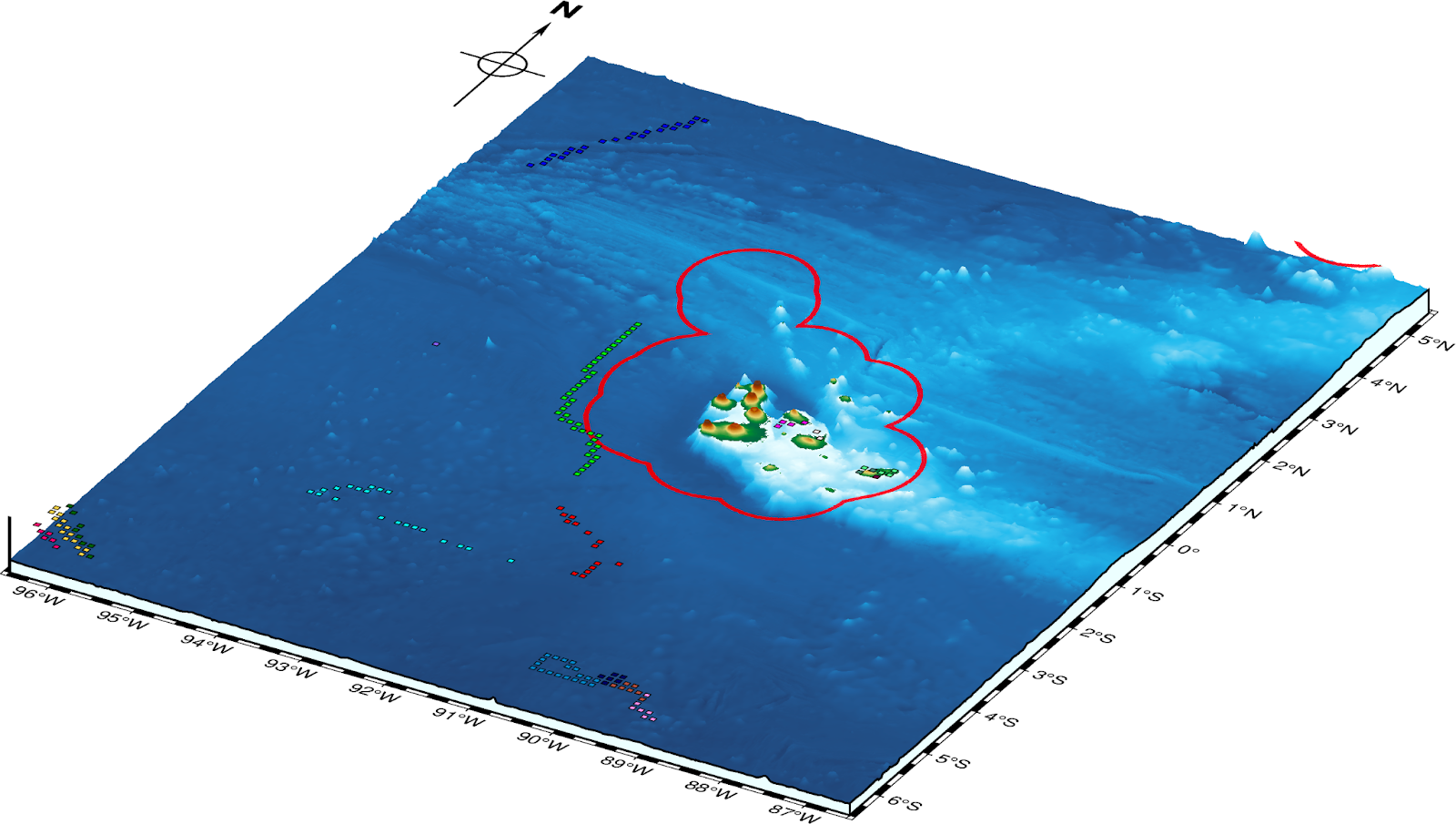
An example of fishing vessels operating near the Galapagos Marine Refuge.
Building on prior work in the literature [1], and fusing data from other sources, such as the HYbrid Coordinate Ocean Model (HYCOM) [2], we will expand our analysis to account for the impact of ocean and atmospheric conditions and currents on the fishing operations and the position of vessels. This approach will help remove false positives, and identify when a vessel is navigating in a way that is out of the norm, perhaps trying to disguise malicious behavior.
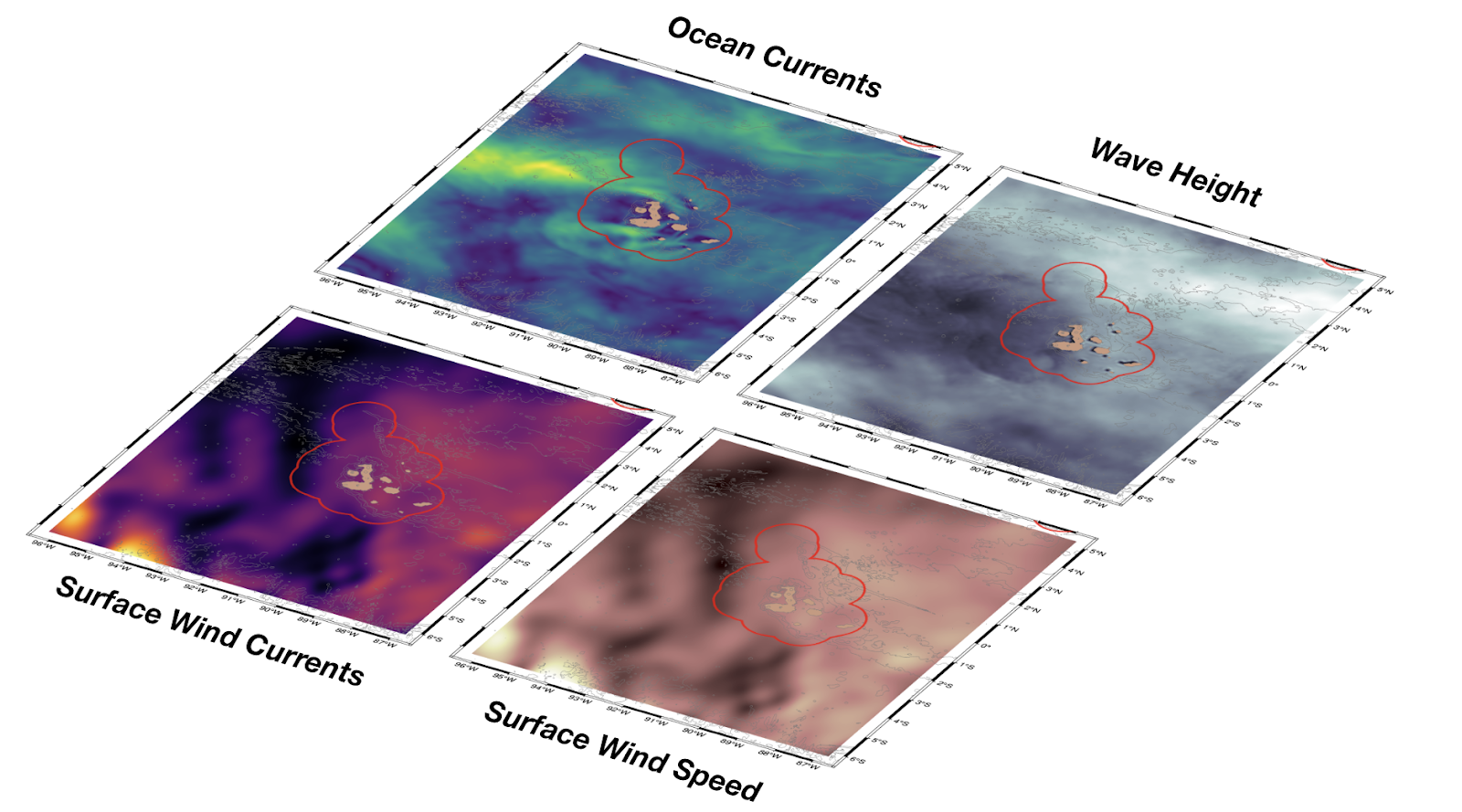
Galois will also be working to include data about the biosphere to better understand where fish populations of various species might be located, leveraging the Modern-Era Retrospective analysis for Research and Applications (MERRA-2) data set [3] and the Moderate Resolution Imaging Spectroradiometer (MODIS) data [4] from NASA’s recent satellite projects to model ocean salinity, temperature, and concentrations of phytoplankton. As shown in the measurement errors present in the salinity and chlorophyll concentration maps, however, the challenge lies not only in data collection but also in its interpretation, integration, and application towards strategic decision-making.
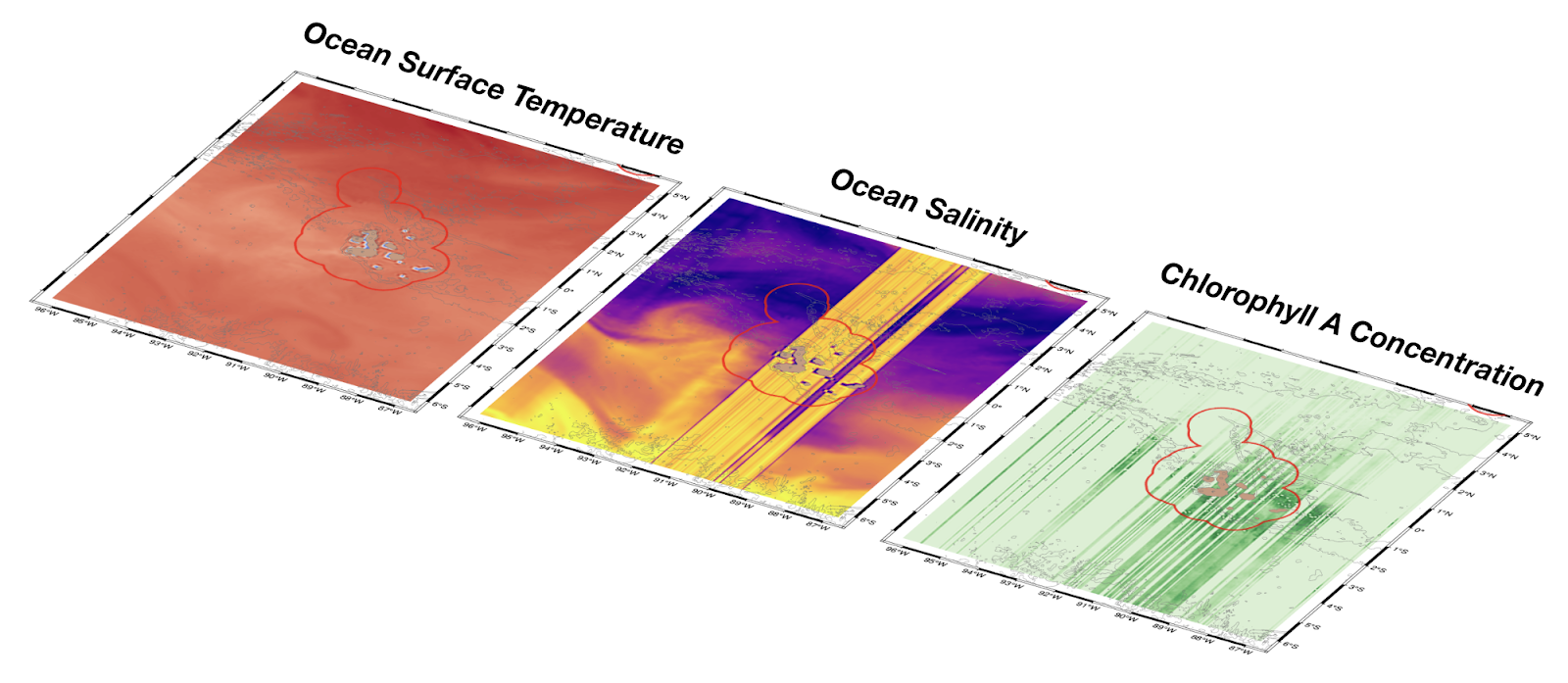
Connecting Dots
This is the promise of AI teammates in this domain: they empower analysts to work with large data sets that cannot be analyzed by humans alone due to their size and scale. All together, the data discussed above form a multidimensional puzzle that human analysts—with help from their AI teammates—can navigate to uncover patterns. Transponder data broadcast from vessels, for example, can help indicate the presence of global fishing vessels in and near protected areas. Suspicious loitering activity, veering off a planned course, or encounters with vessels involved in IUU fishing can indicate the presence of transshipment networks taking on illegal cargo from IUU fishing under the guise of refueling.
Traditional AI teammates require massive amounts of oversight from their human teammates, identifying errors, describing data, and interpreting outcomes. In order to understand the impact of salinity and phytoplankton concentrations shown above, for example, we also have to understand where the data is faulty, and how to deal with diverse sources of error and uncertainty. Our new methods in contextually aware AI will help solve these problems by allowing AI to reason more like a human teammate, requiring less oversight, while still delivering the performance of traditional AI systems.
By analyzing patterns in the data, human-machine teams can connect dots to unveil the shadowy networks that enable this illicit industry, trace their activities, uncover their routes, and anticipate their tactics. Over time, it may be possible to predict the activities of potential perpetrators and interfere before a crime occurs. In tandem with local law enforcement and international organizations like the United Nations and Interpol, this initiative can help eradicate IUU fishing, conserve marine life, and restore the balance in marine ecosystems like the Galapagos Marine Reserve.
To learn more, email us at: contact@galois.com.
REFERENCES
[1] Miller, Nathan A., Aaron Roan, Timothy Hochberg, John Amos, and David A. Kroodsma. “Identifying global patterns of transshipment behavior.” Frontiers in Marine Science 5 (2018): 240.
[2] Funding for the development of HYCOM has been provided by the National Ocean Partnership Program and the Office of Naval Research. Data assimilative products using HYCOM are funded by the U.S. Navy. Computer time was made available by the DoD High Performance Computing Modernization Program. The output is publicly available at https://hycom.org.
[3] Global Modeling and Assimilation Office (GMAO) (2015), inst3_3d_asm_Cp: MERRA-2 3D IAU State, Meteorology Instantaneous 3-hourly (p-coord, 0.625×0.5L42), version 5.12.4, Greenbelt, MD, USA: Goddard Space Flight Center Distributed Active Archive Center (GSFC DAAC)
[4] The Moderate Resolution Imaging Spectroradiometer (MODIS) Normalized Difference Vegetation Index (NDVI) used in this study was processed and produced by the NASA/Goddard Space Flight Center’s Global Inventory Modeling and Mapping Studies (GIMMS) Group through funding support of the Global Agricultural Monitoring project by USDA’s Foreign Agricultural Service (FAS).